Note
Go to the end to download the full example code.
Transport distributions using dense solvers#
In this example, we sample 2 toy distributions and compute a dense fugw alignment between them. Dense alignments are typically used when both aligned distributions have less than 10k points.
import matplotlib.pyplot as plt
import numpy as np
import torch
from fugw.mappings import FUGW
from fugw.utils import _init_mock_distribution
from matplotlib.collections import LineCollection
torch.manual_seed(0)
n_points_source = 50
n_points_target = 40
n_features_train = 2
n_features_test = 2
Let us generate random training data for the source and target distributions
/usr/local/lib/python3.8/site-packages/torch/distributions/wishart.py:272: UserWarning:
Singular sample detected.
torch.Size([2, 50])
We can visualize the generated features:
fig = plt.figure(figsize=(4, 4))
ax = fig.add_subplot()
ax.set_title("Source and target features")
ax.set_aspect("equal", "datalim")
ax.scatter(source_features_train[0], source_features_train[1], label="Source")
ax.scatter(target_features_train[0], target_features_train[1], label="Target")
ax.legend()
plt.show()
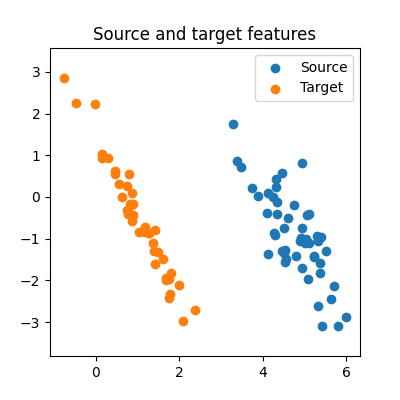
And embeddings:
fig = plt.figure()
ax = fig.add_subplot(projection="3d")
ax.set_title("Source and target embeddings (ie geometries)")
ax.scatter(
source_embeddings[:, 0],
source_embeddings[:, 1],
source_embeddings[:, 2],
s=15,
label="Source",
)
ax.scatter(
target_embeddings[:, 0],
target_embeddings[:, 1],
target_embeddings[:, 2],
s=15,
label="Target",
)
ax.legend()
plt.show()
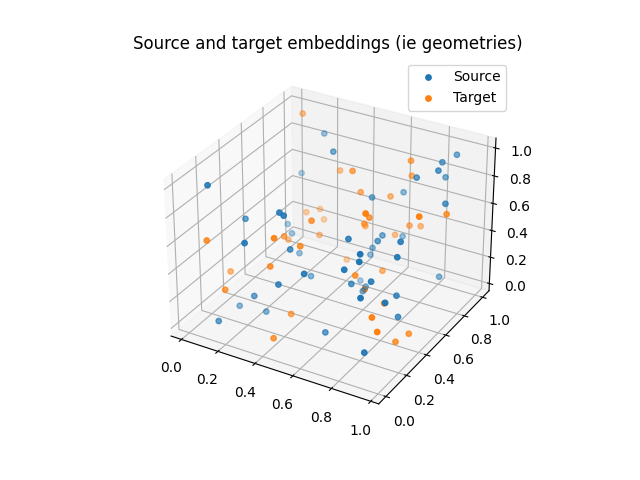
Features and geometries should be normalized before calling the solver
source_features_train_normalized = source_features_train / torch.linalg.norm(
source_features_train, dim=1
).reshape(-1, 1)
target_features_train_normalized = target_features_train / torch.linalg.norm(
target_features_train, dim=1
).reshape(-1, 1)
source_geometry_normalized = source_geometry / source_geometry.max()
target_geometry_normalized = target_geometry / target_geometry.max()
Let us define the optimization problem to solve
Now, we fit a transport plan between source and target distributions using a sinkhorn solver
_ = mapping.fit(
source_features_train_normalized,
target_features_train_normalized,
source_geometry=source_geometry_normalized,
target_geometry=target_geometry_normalized,
solver="sinkhorn",
verbose=True,
)
[14:31:48] Validation data for feature maps is not provided. Using dense.py:199
training data instead.
Validation data for anatomical kernels is not provided. dense.py:226
Using training data instead.
BCD step 1/10 FUGW loss: 0.029136842116713524 dense.py:568
Validation loss: 0.029136842116713524
[14:31:49] BCD step 2/10 FUGW loss: 0.046771109104156494 dense.py:568
Validation loss: 0.046771109104156494
BCD step 3/10 FUGW loss: 0.022854337468743324 dense.py:568
Validation loss: 0.022854337468743324
BCD step 4/10 FUGW loss: 0.02232404239475727 dense.py:568
Validation loss: 0.02232404239475727
[14:31:50] BCD step 5/10 FUGW loss: 0.04798972234129906 dense.py:568
Validation loss: 0.04798972234129906
BCD step 6/10 FUGW loss: 0.02343880571424961 dense.py:568
Validation loss: 0.02343880571424961
BCD step 7/10 FUGW loss: 0.019214436411857605 dense.py:568
Validation loss: 0.019214436411857605
[14:31:51] BCD step 8/10 FUGW loss: 0.01873275265097618 dense.py:568
Validation loss: 0.01873275265097618
BCD step 9/10 FUGW loss: 0.01861358806490898 dense.py:568
Validation loss: 0.01861358806490898
[14:31:52] BCD step 10/10 FUGW loss: 0.018613653257489204 dense.py:568
Validation loss: 0.018613653257489204
The transport plan can be accessed after the model has been fitted
pi = mapping.pi
print(f"Transport plan's total mass: {pi.sum():.5f}")
Transport plan's total mass: 0.99999
Here is the evolution of the FUGW loss during training, as well as the contribution of each loss term:
fig, ax = plt.subplots(figsize=(10, 4))
ax.set_title("Mapping training loss")
ax.set_ylabel("Loss")
ax.set_xlabel("BCD step")
ax.stackplot(
mapping.loss_steps,
[
(1 - alpha) * np.array(mapping.loss["wasserstein"]),
alpha * np.array(mapping.loss["gromov_wasserstein"]),
rho * np.array(mapping.loss["marginal_constraint_dim1"]),
rho * np.array(mapping.loss["marginal_constraint_dim2"]),
eps * np.array(mapping.loss["regularization"]),
],
labels=[
"wasserstein",
"gromov_wasserstein",
"marginal_constraint_dim1",
"marginal_constraint_dim2",
"regularization",
],
alpha=0.8,
)
ax.legend()
plt.show()
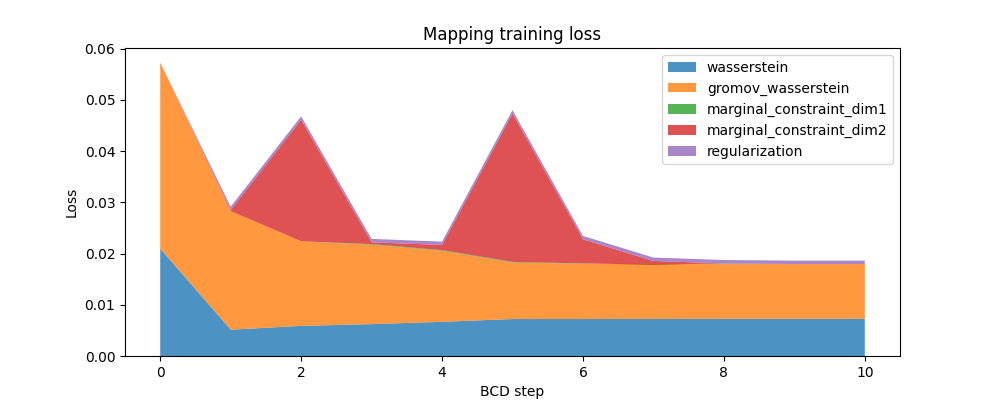
Using the computed mapping#
The computed mapping is stored in mapping.pi
as a torch.Tensor
.
In this example, the transport plan is small enough that we can display
it altogether.
fig, ax = plt.subplots(figsize=(4, 4))
ax.set_title("Transport plan")
ax.set_xlabel("target vertices")
ax.set_ylabel("source vertices")
im = plt.imshow(pi, cmap="viridis")
plt.colorbar(im, ax=ax, shrink=0.8)
plt.show()
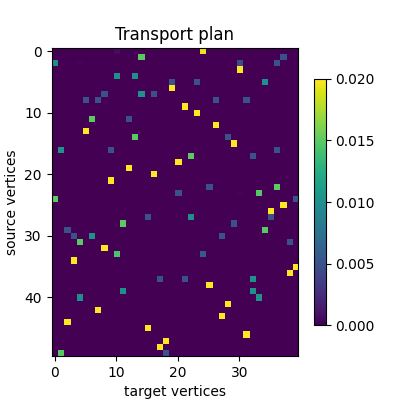
The previous figure of the transport plan tells us it is very sparse and not very regularized. Another informative way to look at the plan consists in checking which points of the source and target distributions were matched together in the feature space.
fig = plt.figure(figsize=(4, 4))
ax = fig.add_subplot()
ax.set_aspect("equal", "datalim")
ax.set_title("Mapping\ndisplayed in feature space")
# Draw lines between matched points
indices = torch.cartesian_prod(
torch.arange(n_points_source), torch.arange(n_points_target)
)
segments = torch.stack(
[
source_features_train[:, indices[:, 0]],
target_features_train[:, indices[:, 1]],
]
).permute(2, 0, 1)
pi_normalized = pi / pi.sum(dim=1).reshape(-1, 1)
line_segments = LineCollection(
segments, alpha=pi_normalized.flatten(), colors="black", lw=1, zorder=1
)
ax.add_collection(line_segments)
# Draw distributions
ax.scatter(source_features_train[0], source_features_train[1], label="Source")
ax.scatter(target_features_train[0], target_features_train[1], label="Target")
ax.legend()
plt.show()
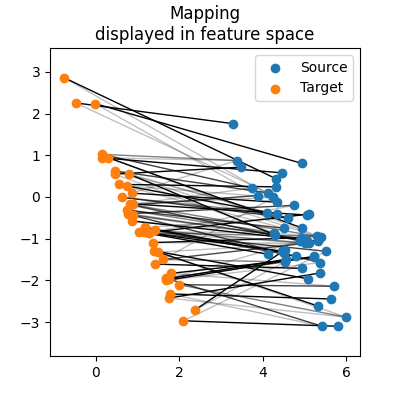
Finally, the fitted model can transport unseen data between source and target
source_features_test = torch.rand(n_features_test, n_points_source)
target_features_test = torch.rand(n_features_test, n_points_target)
transformed_data = mapping.transform(source_features_test)
transformed_data.shape
torch.Size([2, 40])
assert transformed_data.shape == target_features_test.shape
Total running time of the script: (0 minutes 6.388 seconds)
Estimated memory usage: 134 MB