Note
Go to the end to download the full example code.
Low-resolution volume alignment of 2 individuals with fMRI data#
In this example, we align 2 low-resolution brain volumes using 4 fMRI feature maps (z-score contrast maps).
import numpy as np
import matplotlib.colors as colors
import matplotlib.pyplot as plt
from mpl_toolkits.axes_grid1 import make_axes_locatable
from nilearn import datasets, image
from scipy.spatial import distance_matrix
from fugw.mappings import FUGW
We first fetch 5 contrasts for each subject from the localizer dataset.
n_subjects = 2
contrasts = [
"sentence reading vs checkerboard",
"sentence listening",
"calculation vs sentences",
"left vs right button press",
"checkerboard",
]
n_training_contrasts = 4
brain_data = datasets.fetch_localizer_contrasts(
contrasts,
n_subjects=n_subjects,
get_anats=True,
)
source_imgs_paths = brain_data["cmaps"][0 : len(contrasts)]
target_imgs_paths = brain_data["cmaps"][len(contrasts) : 2 * len(contrasts)]
source_im = image.load_img(source_imgs_paths)
target_im = image.load_img(target_imgs_paths)
We greatly downsample all image to reduce the computational cost so that this example will run on a CPU.
SCALE_FACTOR = 5
source_maps = np.nan_to_num(
source_im.get_fdata()[::SCALE_FACTOR, ::SCALE_FACTOR, ::SCALE_FACTOR]
)
target_maps = np.nan_to_num(
target_im.get_fdata()[::SCALE_FACTOR, ::SCALE_FACTOR, ::SCALE_FACTOR]
)
segmentation_fine = np.logical_not(np.isnan(source_im.get_fdata()[:, :, :, 0]))
segmentation_coarse = segmentation_fine[
::SCALE_FACTOR, ::SCALE_FACTOR, ::SCALE_FACTOR
]
coordinates = np.array(np.nonzero(segmentation_coarse)).T
fig = plt.figure(figsize=(5, 5))
ax = fig.add_subplot(projection="3d")
ax.set_title("Anatomical coordinates of voxels")
ax.scatter(coordinates[:, 0], coordinates[:, 1], coordinates[:, 2], marker="o")
ax.view_init(10, 135)
ax.set_axis_off()
plt.show()
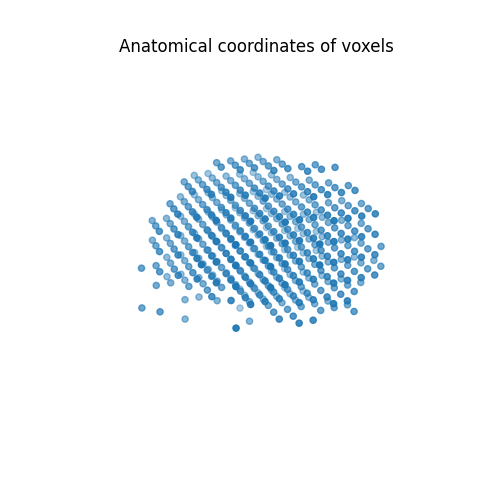
Eventually, we extract the features at each voxel and end up with roughly 500 voxels.
source_features = source_maps[
coordinates[:, 0], coordinates[:, 1], coordinates[:, 2]
].T
target_features = target_maps[
coordinates[:, 0], coordinates[:, 1], coordinates[:, 2]
].T
source_features.shape
(5, 484)
We then compute the distance matrix between voxel coordinates.
source_geometry = distance_matrix(coordinates, coordinates)
target_geometry = source_geometry.copy()
fig = plt.figure(figsize=(5, 5))
ax = fig.add_subplot(111)
ax.set_title("Distance matrix between voxels")
im = ax.imshow(source_geometry)
divider = make_axes_locatable(ax)
cax = divider.append_axes("right", size="3%", pad="2%")
fig.colorbar(im, cax=cax, label="mm")
plt.tight_layout()
plt.show()
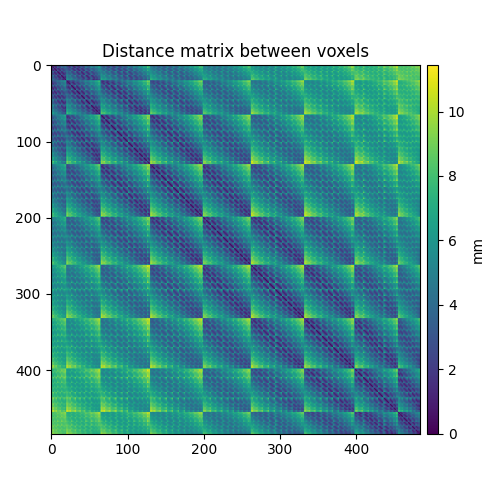
vertex_index = 4
fig = plt.figure(figsize=(7, 5))
ax = fig.add_subplot(111, projection="3d")
ax.set_title(f"Distance to source voxel {vertex_index}")
# Plot brain geometry
im = ax.scatter(
coordinates[1:, 0],
coordinates[1:, 1],
coordinates[1:, 2],
marker=".",
c=source_geometry[0, 1:],
)
# Add source point label
ax.scatter(
coordinates[vertex_index, 0],
coordinates[vertex_index, 1],
coordinates[vertex_index, 2],
marker="o",
c="black",
)
ax.text(
coordinates[vertex_index, 0],
coordinates[vertex_index, 1],
coordinates[vertex_index, 2] - 2,
"Source point",
size=12,
color="black",
)
# Add colorbar
colorbar = fig.colorbar(
im,
ax=ax,
label="mm",
)
colorbar.ax.set_position([0.9, 0.15, 0.03, 0.7])
ax.view_init(10, 135)
ax.set_axis_off()
plt.tight_layout()
plt.show()
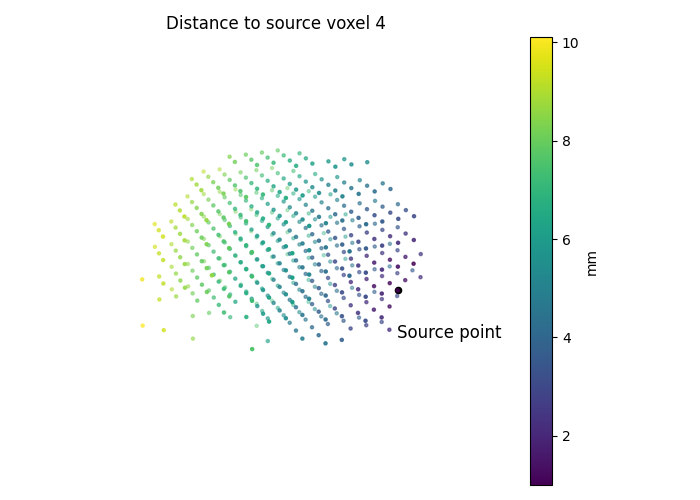
In order to avoid numerical errors when fitting the mapping, we normalize both the features and the geometry.
source_features_normalized = source_features / np.linalg.norm(
source_features, axis=1
).reshape(-1, 1)
target_features_normalized = target_features / np.linalg.norm(
target_features, axis=1
).reshape(-1, 1)
source_geometry_normalized = source_geometry / np.max(source_geometry)
target_geometry_normalized = target_geometry / np.max(target_geometry)
We now fit the mapping using the sinkhorn solver and 3 BCD iterations.
mapping = FUGW(alpha=0.5, rho=1, eps=1e-4)
_ = mapping.fit(
source_features_normalized[:n_training_contrasts],
target_features_normalized[:n_training_contrasts],
source_geometry=source_geometry_normalized,
target_geometry=target_geometry_normalized,
solver="sinkhorn",
solver_params={
"nits_bcd": 4,
},
verbose=True,
)
[14:37:01] Validation data for feature maps is not provided. Using dense.py:199
training data instead.
Validation data for anatomical kernels is not provided. dense.py:226
Using training data instead.
[14:37:07] BCD step 1/4 FUGW loss: 0.025816654786467552 dense.py:568
Validation loss: 0.025816654786467552
[14:37:17] BCD step 2/4 FUGW loss: 0.009248202666640282 dense.py:568
Validation loss: 0.009248202666640282
[14:37:26] BCD step 3/4 FUGW loss: 0.007908511906862259 dense.py:568
Validation loss: 0.007908511906862259
[14:37:35] BCD step 4/4 FUGW loss: 0.007553447037935257 dense.py:568
Validation loss: 0.007553447037935257
Let’s plot the probability map of target voxels being matched with the 4th source voxel.
pi = mapping.pi
probability_map = pi[vertex_index, :] / np.sqrt(
np.linalg.norm(pi[vertex_index, :])
)
fig = plt.figure(figsize=(5, 5))
ax = fig.add_subplot(projection="3d")
ax.set_title(
"Probability map of target voxels\n"
f"being matched with source voxel {vertex_index}"
)
s = ax.scatter(
coordinates[:, 0],
coordinates[:, 1],
coordinates[:, 2],
marker=".",
c=probability_map,
cmap="viridis",
alpha=0.75,
)
colorbar = fig.colorbar(s, ax=ax)
colorbar.ax.set_position([0.9, 0.15, 0.03, 0.7])
ax.view_init(10, 135, 2)
ax.set_axis_off()
plt.tight_layout()
plt.show()
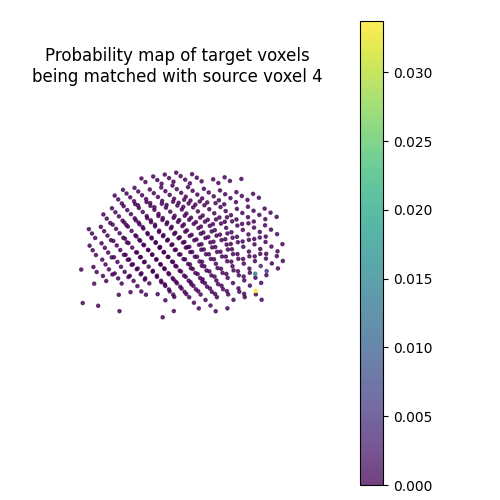
We can now align test contrasts using the fitted mapping.
contrast_index = -1
predicted_target_features = mapping.transform(
source_features[contrast_index, :]
)
predicted_target_features.shape
(484,)
Let’s compare the Pearson correlation between source and target features.
corr_pre_mapping = np.corrcoef(
source_features[contrast_index, :], target_features[contrast_index, :]
)[0, 1]
corr_post_mapping = np.corrcoef(
predicted_target_features, target_features[contrast_index, :]
)[0, 1]
print(f"Pearson Correlation pre-mapping: {corr_pre_mapping:.2f}")
print(f"Pearson Correlation post-mapping: {corr_post_mapping:.2f}")
print(
"Relative improvement:"
f" {(corr_post_mapping - corr_pre_mapping) / corr_pre_mapping * 100 :.2f}"
" %"
)
Pearson Correlation pre-mapping: 0.28
Pearson Correlation post-mapping: 0.37
Relative improvement: 30.13 %
Let’s plot the transporting feature maps of the test set.
fig = plt.figure(figsize=(12, 4))
fig.suptitle("Transporting feature maps of the test set")
ax = fig.add_subplot(1, 3, 1, projection="3d")
s = ax.scatter(
coordinates[:, 0],
coordinates[:, 1],
coordinates[:, 2],
marker="o",
c=source_features_normalized[-1, :],
cmap="coolwarm",
norm=colors.CenteredNorm(),
)
ax.view_init(10, 135, 2)
ax.set_title("Source features")
ax.set_axis_off()
ax = fig.add_subplot(1, 3, 2, projection="3d")
ax.scatter(
coordinates[:, 0],
coordinates[:, 1],
coordinates[:, 2],
marker="o",
c=predicted_target_features,
cmap="coolwarm",
norm=colors.CenteredNorm(),
)
ax.view_init(10, 135, 2)
ax.set_title("Predicted target features")
ax.set_axis_off()
ax = fig.add_subplot(1, 3, 3, projection="3d")
ax.scatter(
coordinates[:, 0],
coordinates[:, 1],
coordinates[:, 2],
marker="o",
c=target_features_normalized[-1, :],
cmap="coolwarm",
norm=colors.CenteredNorm(),
)
ax.view_init(10, 135, 2)
ax.set_title("Actual target features")
ax.set_axis_off()
ax = fig.add_subplot(1, 1, 1)
ax.set_axis_off()
divider = make_axes_locatable(ax)
cax = divider.append_axes("right", size="1%")
fig.colorbar(s, cax=cax)
plt.tight_layout()
plt.show()
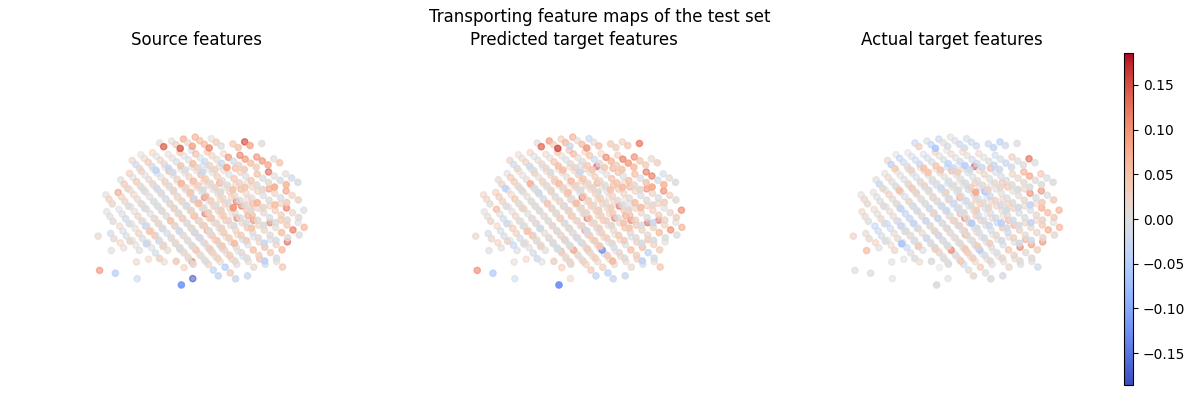
Total running time of the script: (0 minutes 38.479 seconds)
Estimated memory usage: 65 MB