Note
Go to the end to download the full example code.
Generate embeddings from mesh#
In this example, we show how to derive an embedding which approximates the kernel matrix of geodesic distances on a given mesh. This technique is useful when trying to align distributions with a large number of points. Indeed, the kernel matrix of pairwise distances won’t fit in memory, but an embedding computed in the right dimension can probably estimate it.
import gdist
import matplotlib.pyplot as plt
import numpy as np
import torch
from fugw.scripts import lmds
from nilearn import datasets, surface
Here, we will compute the exact geodesic distances from
each vertex to a random sample of n_landmarks
vertices.
The derived embedding will be in dimension k
.
torch.manual_seed(0)
n_landmarks = 100
k = 3
Let us load a pre-computed mesh and have a look at it first
fsaverage3 = datasets.fetch_surf_fsaverage(mesh="fsaverage3")
coordinates, triangles = surface.load_surf_mesh(fsaverage3.sphere_left)
coordinates.shape
Added README.md to /github/home/nilearn_data
Dataset created in /github/home/nilearn_data/fsaverage3
Downloading data from https://osf.io/azhdf/download ...
...done. (2 seconds, 0 min)
Extracting data from /github/home/nilearn_data/fsaverage3/472ead7320dbd53fb2ec42e05f1bb6bf/download..... done.
(642, 3)
fig = plt.figure(figsize=(5, 5))
ax = fig.add_subplot(projection="3d")
ax.plot_trisurf(
coordinates[:, 0],
coordinates[:, 1],
coordinates[:, 2],
triangles=triangles,
)
plt.show()
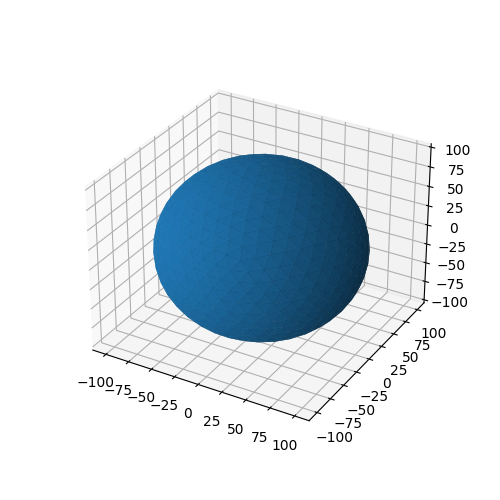
Now, let’s compute the embedding! This computation is easy to parallelize.
X = lmds.compute_lmds_mesh(
coordinates,
triangles,
n_landmarks=n_landmarks,
k=k,
n_jobs=2,
verbose=True,
)
100% Geodesic distances (landmarks) ━━━━━━━━━━━━━━━━ 100/100 0:00:02 < 0:00:00
/github/workspace/src/fugw/scripts/lmds.py:175: UserWarning:
A might not be centered (1.8671875 > 0.001)
It should have the correct size
assert X.shape == (coordinates.shape[0], k)
We can actually have a peek at the computed embedding:
fig = plt.figure(figsize=(5, 5))
ax = fig.add_subplot(projection="3d")
ax.set_title("Embedding approximating kernel matrix")
ax.scatter(
X[:, 0],
X[:, 1],
X[:, 2],
s=15,
)
plt.show()
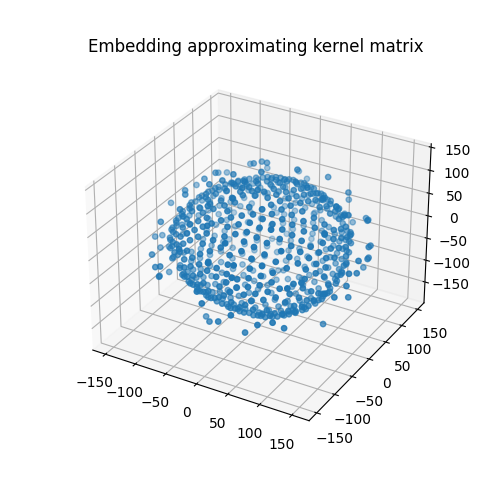
Finally, we check that the exact matrix of geodesic distances between pairs of vertices of the mesh is well approximated by the kernel matrix derived from the embeddings:
fig = plt.figure(figsize=(5, 10))
ax = fig.add_subplot(211)
ax.set_title("True matrix of geodesic distances")
true_kernel_matrix = gdist.local_gdist_matrix(
coordinates.astype(np.float64),
triangles.astype(np.int32),
).toarray()
im = ax.imshow(true_kernel_matrix)
plt.colorbar(im, ax=ax, shrink=0.9)
ax = fig.add_subplot(212)
ax.set_title("Approximated matrix of geodesic distances")
approximated_kernel_matrix = torch.cdist(X, X)
im = ax.imshow(approximated_kernel_matrix)
plt.colorbar(im, ax=ax, shrink=0.9)
plt.show()
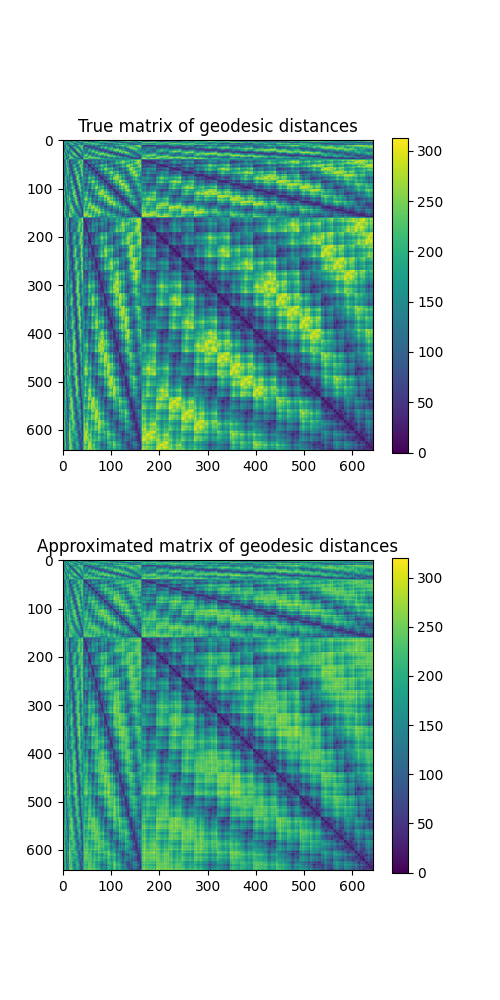
Total running time of the script: (0 minutes 8.929 seconds)
Estimated memory usage: 138 MB